The current state of AI: the trough of disillusionment
Expectation: “AI is here!”🚀
Reality: 👀
Fifty-six percent of companies say “inaccuracy” is the biggest risk posed by adopting generative AI
– MCKINSEY, 2023
We are in the depths of what Gartner refers to as the trough of disillusionment in their hype cycle.
This is one of five key phases in a technology life cycle where interest decreases due to experiments and implementations failing to deliver. Additionally, investments in technology only continue if the surviving producers improve their products.

The bottom line is that the hype is real and the rise of AI is putting new pressures on businesses.
Gartner says “45% of leaders reported that the recent hype around ChatGPT prompted them to increase AI investments.”
Additionally, 70% of leaders say their organization is investigating and exploring generative AI, while 19% are in pilot or production mode.
Yet we’re not quite “there” — yet.
With new technology comes new datasets, processes, and governance. Organizations first need to focus on getting their data foundations right to prepare for when AI begins to trend upward toward the slope of enlightenment (and it will).
Here’s why.
Reason 1: Garbage in equals garbage out. Without clean, connected, accurate data, AI will steer you in the wrong direction.
Reason 2: People + process. Without governance, AI can’t effectively govern your team.
The takeaway? While AI is hungry for insights, it also doesn’t care about what it feeds on. If you’re fueling AI with bad data, guess what you’ll get? Garbage in…garbage out.
So before starting your next AI project, build the right foundation to get the best results for your business.
How to prepare for AI today
1. Establish Data Quality Metrics
Before improving data quality, you need to understand what that means to your organization. Define clear, measurable metrics around data quality your company wants to prioritize, such as accuracy, completeness, consistency, timeliness, and uniqueness.
These metrics are benchmarks for assessing your data’s current state and tracking improvements over time.
2. Conduct a data quality audit
A comprehensive data audit will help you identify the strengths and weaknesses in your current data ecosystem. Evaluate your data sources, storage, and management practices against the data quality metrics you’ve established. Look for common issues that are hindering accessibility and integration, such as duplicate records, outdated information, or data silos.
Use account hierarchy automation to identify duplicate account records and seamlessly connect related accounts rather than proliferate dupes.
3. Automate data cleanup
Based on the findings from your audit, develop and implement processes for cleaning your data. This can include proactive and reactive deduplication, correcting errors, and filling in missing values.
Data cleansing should also be treated as an ongoing process, as opposed to a one-time project, with regular reviews to ensure that data quality is maintained as your business and AI projects grow.
Tools like Complete Clean automate the reactive data cleanup process without losing trust in the output:
- Use different merge plans with different duplicate rules associated
- Run multiple duplicate definitions/rules
- Put no limit on the number of duplicates you can run
Proactive data cleanup can also be done with lead-to-account matching upon entry so you don’t lose critical insights or block duplicates with more relevant information from being created.
4. Standardize data entry and collection
Inconsistencies around data collection and entry can lead to significant data quality issues. That’s why you need to establish standardized procedures and formatting for data entry across your organization. This can include standard forms, validation rules to prevent errors at the point of entry, and training for staff on the importance of data quality.
Tactical tips include:
- Ensuring that all forms include mandatory fields that must be filled out (ensuring data completeness)
- Using picklists instead of free-text fields to limit variations in data entry
- Creating validation rules that automatically check the data being entered against specific criteria
When creating validation rules, you can set up rules that verify that email addresses are in the correct format or that mandatory fields aren’t left black. This helps maintain the integrity and accuracy of the data being entered.
5. Implement data governance
Data quality should be a shared responsibility across the organization, not just a concern of the IT department or data specialists. That’s why it’s important to build a culture that recognizes the value of quality data and encourages best practices in data management.
Regular training sessions, clear communication of data quality standards, and recognition of teams that maintain high data quality all contribute to this culture.
Define your dimensions and establish specific, measurable metrics for data quality. For example:
- Accuracy: Percentage of records that pass data validation rules
- Completeness: Percentage of mandatory fields filled in a dataset
- Consistency: Number of discrepancies found between related datasets
- Timeliness: Average age of data records or time taken to update data after changes
- Uniqueness: Percentage of unique records in a dataset, with no duplicates
6. Monitor and maintain data quality
Continous attention is essential to keeping data quality. Set up systems and processes to regularly assess your data against the quality metrics you’ve established. This helps you catch and address issues quickly, before they impact your AI initiatives.
Regularly review and update your data management practices to adapt to new challenges and opportunities.
7. Prepare for integration
AI often needs data integration from multiple sources. Prepare your data for this reality by ensuring it’s structured and formatted in a way that supports integration. This might involve standardizing data formats, developing a data warehouse or lake, and establishing protocols for data sharing.
On top of that, clearly define what you hope to achieve with data integration, whether it’s improving data quality, enabling real-time analytics, or feeding a unified data set into an AI model for better predictions. Agree on common data formats, naming conventions, and structures.
Get the AI readiness checklist for revenue teams
Ready to get started on your AI project? Create a checklist and communicate to your team what needs to happen before any AI implementation:
- Centralized data across disconnected systems
- Automation flows of data between teams, tools, and platforms
- A process for identifying and removing duplicate records
- Processes and milestones for continual data quality improvement
- Clear data governance practices
- Business objectives of AI initiatives and expected outcomes
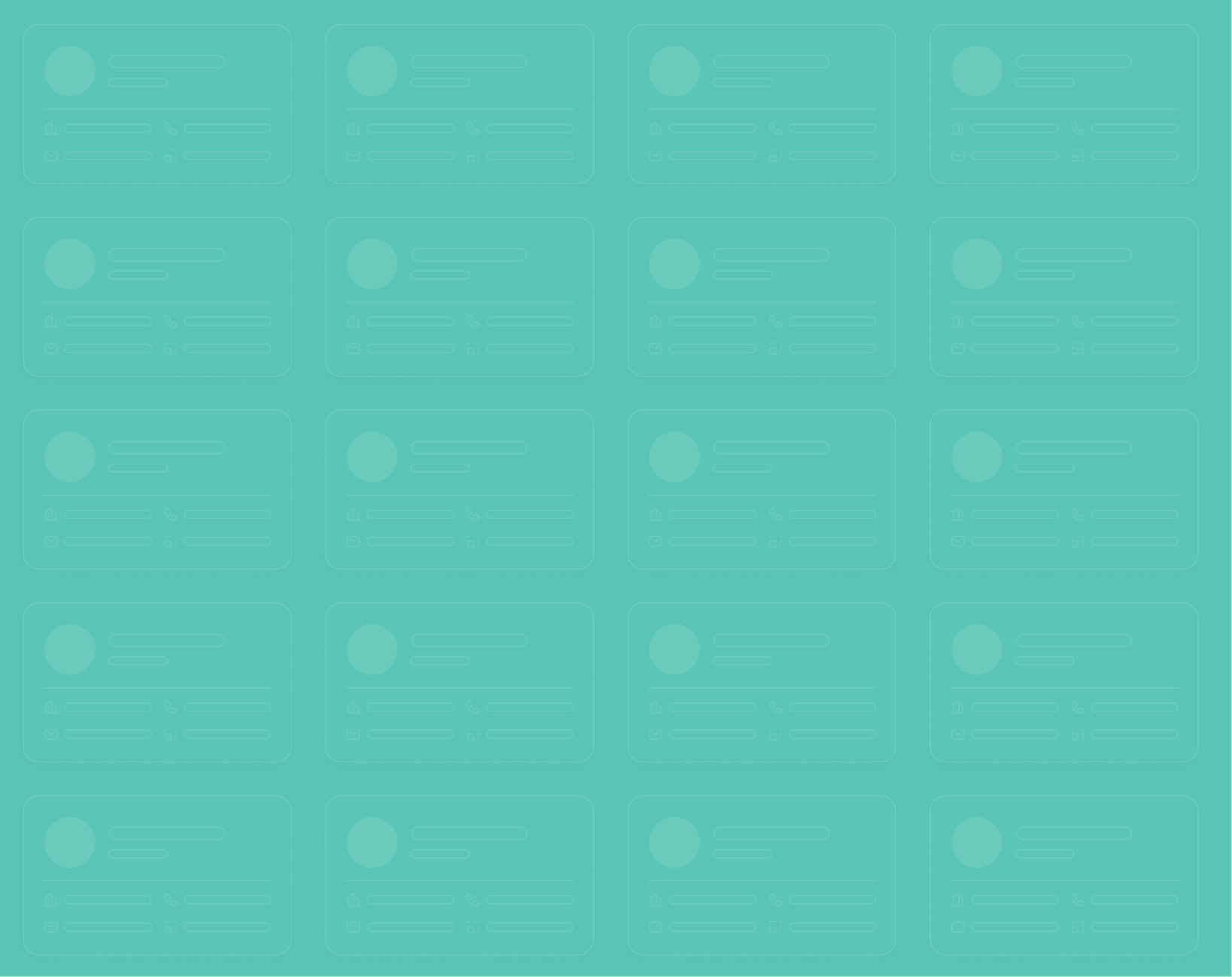